Understanding Historical Sales Forecasting

Sales teams have long relied on historical data to predict future performance. By analyzing past sales records, businesses can spot important patterns and make smarter decisions about inventory, resources, and strategy.
Why Historical Data Matters
Past performance gives you reliable clues about what to expect, especially when market conditions are stable. For instance, if your sales have grown steadily by 10% each quarter for the past two years, that trend likely indicates continued growth ahead. Similarly, predictable seasonal spikes help you plan for busy periods.
The beauty of historical forecasting lies in its simplicity. For example, if your monthly sales averaged $100,000 last year and you project 10% growth, you can reasonably expect $110,000 next month. This straightforward approach works particularly well in stable markets where past patterns tend to repeat. However, it may miss sudden changes or new product launches that could impact future sales. Learn more about sales forecasting methods here.
Identifying Key Patterns
Good historical forecasting goes beyond just copying last year's numbers. The real value comes from understanding what drives sales performance - whether that's seasonal demand, marketing campaigns, or product lifecycles. A clothing retailer, for example, might notice that winter coats consistently sell best in October, helping them time inventory purchases just right.
The Role of Historical Forecasting in a Modern Context
While new AI and machine learning tools are changing how we forecast sales, historical data remains essential. Past performance creates a baseline for measuring progress and validating newer forecasting models. Many modern systems actually combine traditional historical analysis with advanced algorithms to produce more accurate predictions. This hybrid approach helps businesses make better decisions by leveraging both time-tested methods and new technology.
Advanced Time Series and Consumption-Based Approaches
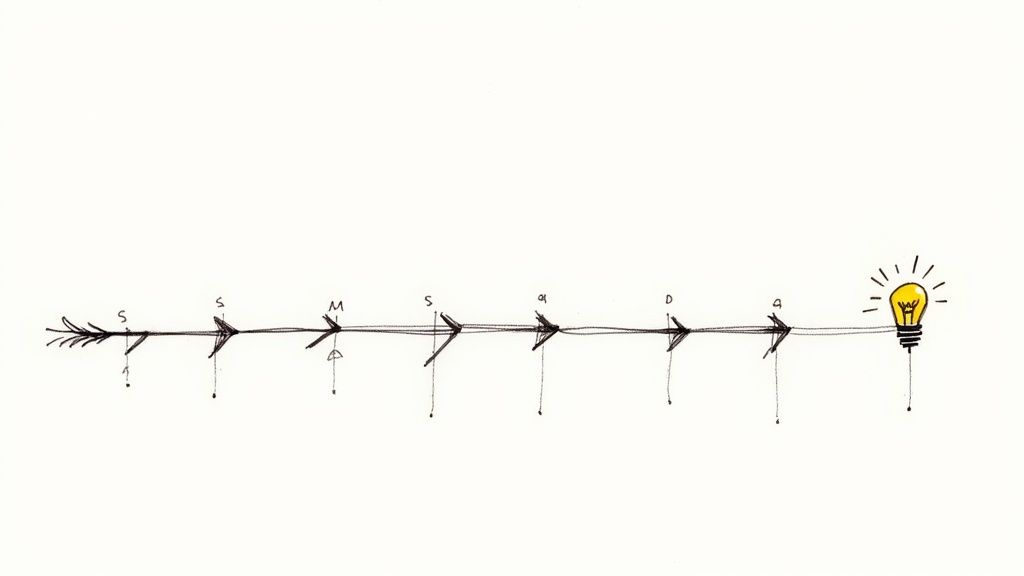
Smart sales forecasting requires looking beyond basic historical data analysis. By using advanced techniques like time series analysis and consumption-based forecasting, businesses can predict future sales with remarkable accuracy. Let's explore how these methods work and help drive better revenue predictability.
Time Series Analysis: Understanding Patterns Over Time
Time series analysis helps businesses spot important trends by studying data over specific time periods. This is especially valuable for companies with seasonal sales patterns. For example, a clothing retailer can analyze past winter coat sales to predict demand for the upcoming cold season.
By identifying recurring patterns, companies can plan inventory, marketing, and staffing more effectively. Time series analysis also factors in external influences like economic indicators and promotional campaigns. This gives businesses deeper insight into what drives sales fluctuations over time.
Consumption-Based Forecasting: Tracking Usage in Subscription Models
For subscription-based businesses, understanding how customers use their products is key to predicting future sales. Consumption-based forecasting analyzes past usage data to anticipate customer behavior and demand. For instance, a streaming service can track viewing habits to predict upgrade rates.
This approach lets companies make smarter decisions about resource planning and targeted promotions. It also provides more accurate projections of recurring revenue and customer lifetime value.
Choosing the Right Approach: Matching Methods to Your Needs
The best forecasting method depends on your specific business model and available data. Time series analysis works well when you have clear seasonal patterns, while consumption-based forecasting makes sense for subscription businesses tracking usage.
Historical forecasting breaks down into several approaches, each suited for different needs. Time series analysis focuses on specific periods and helps with seasonal predictions. Consumption-based methods use subscription data to project future sales and usage. While these approaches work well in stable markets, they may miss unexpected changes. Learn more about sales forecasting methods.
The most effective strategy often combines multiple approaches tailored to your unique situation. By understanding the strengths of each method, you can build a forecasting system that delivers reliable predictions to guide business decisions.
Best Practices for Historical Data Analysis
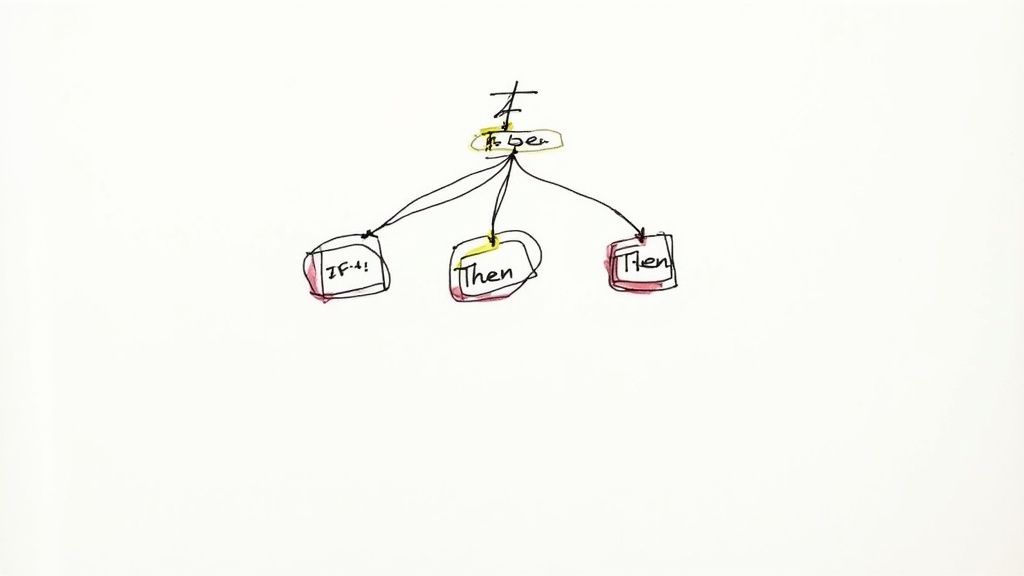
Making sense of past sales data requires more than just looking at numbers. You need a structured approach that focuses on real patterns while accounting for market changes. This section explores proven methods that successful companies use to analyze historical data for better sales forecasting.
Identifying Meaningful Patterns and Filtering Out Noise
The first step is learning to spot true trends versus random fluctuations. For instance, a temporary sales bump from a one-off promotion won't help predict future performance. Instead, focus on consistent patterns like seasonal cycles or steady growth rates that repeat over time. Take note of external factors too - major economic shifts or competitor moves can explain past results and inform future predictions.
Setting Optimal Timeframes for Analysis
The time period you analyze directly impacts forecast accuracy. Looking at too short a timeframe misses bigger trends, while going too far back includes outdated data. A good rule of thumb is to analyze at least twice the length of time you want to forecast. For example, to predict next quarter's sales, examine data from the past two quarters minimum. This helps catch relevant cycles and patterns. Remember though - history rarely repeats exactly, so some adjustments are usually needed. Learn more about sales forecasting approaches.
Adjusting Historical Data to Reflect Current Market Conditions
Markets are always changing. Customer preferences shift, new competitors emerge, and economic conditions evolve. Simply extending past trends won't create accurate forecasts. You need to adjust historical data based on current realities. For example, if a strong new competitor entered your market, you may need more conservative growth projections. Similarly, changing consumer behaviors might require updates to your product demand forecasts. Staying flexible and adapting your analysis helps create reliable sales predictions.
Leveraging Technology in Sales Forecasting

Technology has fundamentally changed how businesses predict their future sales numbers. Modern tools allow companies to make more accurate forecasts and quickly adapt to market changes. Let's explore how Artificial Intelligence (AI), Machine Learning (ML), and advanced data analytics help companies forecast sales more effectively.
The Power of AI and Machine Learning
AI and ML solutions shine at processing massive datasets to spot hidden patterns and make highly accurate predictions. These technologies can analyze far more information than humans - from historical sales data to market trends, economic indicators, and social media sentiment. For instance, AI can detect subtle connections between marketing campaigns and sales increases that traditional analysis might overlook. This deeper insight helps teams optimize their strategies and use resources more wisely. As market conditions shift, the AI continuously learns and updates its forecasts to stay accurate.
Advanced Analytics: Unlocking Deeper Insights
Modern analytics tools give sales teams an in-depth view of what drives their performance. They break down customer data into segments, track important metrics, and generate custom reports. Teams can see which customer groups are most likely to buy, what products have the highest demand, and how outside factors affect purchasing. This detailed understanding lets businesses create targeted promotions for specific segments to boost conversion rates. With this level of insight, companies can stay ahead by proactively adjusting their sales approach.
Implementing Technology Effectively
Adding new technology to existing sales processes requires careful planning. Training is essential - teams need to understand not just how to use the tools, but how to apply the insights they provide. For example, sales reps must learn to translate AI predictions into practical sales strategies and quota planning. Each business also needs to choose solutions that match their size and industry. A small company may not need the same complex system as a large enterprise. Traditional forecasting methods still have value too, especially in stable markets. Over 200 major companies have seen strong returns from investing in forecasting systems that combine historical and new data analysis. In the 1980s, IBM's strong growth came partly from effective sales forecasting that balanced historical trends with emerging insights. The best approach often combines proven methods with new technology to get the most accurate and useful predictions.
Combining Multiple Forecasting Methods
Getting accurate sales predictions requires more than just one forecasting approach. Smart businesses use multiple methods together to get the best results. Each method has its strengths - for example, analyzing past data provides concrete evidence, while expert opinions offer valuable context about where markets are heading. By blending different approaches thoughtfully, companies can build forecasts that are both grounded in data and responsive to changing conditions.
Balancing Qualitative and Quantitative Approaches
The most successful companies use both qualitative and quantitative methods in their forecasting. Looking at past performance through time series analysis gives you hard numbers to work with. But you also need qualitative input from market research and industry experts to understand customer trends and competitive shifts. For example, your historical data might show steady growth, but market experts could alert you to new competitors or changing customer preferences that could impact future sales.
Frameworks for Combining Methods
One practical way to blend methods is to weight different forecasts based on how reliable they are in your specific situation. In stable markets, you might rely more heavily on historical patterns. But when things are changing rapidly, you may want to put more weight on qualitative insights and leading indicators. Another approach is using one primary method and cross-checking it against secondary methods. You should also consider what you're forecasting - new products with no sales history need more emphasis on market research and competitive analysis.
Real-World Examples of Combined Forecasting
Many leading companies now use a mix of historical analysis, market intelligence, customer data, and technology to build stronger forecasting systems. Take a software company that combines past sales data with usage patterns and customer feedback to predict churn rates and future revenue. This gives them a fuller picture by looking at both historical performance and customer behavior signals. Companies are also pulling in data from their CRM systems, marketing platforms, and external research to see all the factors that could affect sales.
Adapting Your Approach to Market Conditions
Markets rarely stay the same for long, so your forecasting needs to be flexible. This means regularly assessing how well different methods are working and adjusting their emphasis based on current conditions. During economic uncertainty, you may need to rely less on historical trends and more on real-time market signals. New competition or technology shifts might require adding different data sources to maintain accuracy. By staying nimble in your approach, you can keep your sales forecasting relevant and reliable even as markets evolve.
Implementing and Maintaining Your Forecasting Strategy
A solid forecasting strategy goes beyond just selecting methods - it requires careful planning, consistent execution, and ongoing refinement. To make sales forecasting work reliably over time, you need robust processes, well-trained teams, and regular performance reviews. Here's how to build a forecasting system that consistently delivers results.
Building Effective Data Collection Processes
Quality data forms the foundation of accurate forecasting. Start by mapping out your key data sources, including sales records, CRM data, website analytics, and market research. Focus on keeping data clean, organized and consistent across platforms. For example, connect your CRM directly to accounting software to reduce manual entry errors. The better your data inputs, the more reliable your forecasts will be.
Training Your Team for Consistent Execution
Even the best forecasting methods only work when used properly. Take time to thoroughly train your sales and marketing teams through workshops, tutorials, and hands-on practice. Emphasize the importance of consistent data entry and following established procedures. Schedule regular refresher sessions to maintain standards and address any challenges that come up.
Establishing Regular Review Procedures
Successful forecasting requires ongoing monitoring and adjustment. Set up monthly or quarterly review meetings to assess forecast accuracy and identify improvement areas. For example, compare actual versus predicted sales figures and investigate any major discrepancies. This allows you to spot issues early and refine your approach based on real performance data.
Gaining Organizational Buy-In and Managing Change
New forecasting approaches often mean workflow changes, which can face resistance. Get ahead of this by clearly communicating benefits to all stakeholders - how better forecasting will improve decision-making and impact different roles. Address concerns openly and provide support during the transition. Highlight early wins and positive metrics to build confidence in the new system.
Measuring Forecast Accuracy and Making Improvements
Track key metrics like Mean Absolute Percentage Error (MAPE) and Weighted MAPE to gauge how well your forecasts perform. Use this data to pinpoint areas needing improvement, whether that's data quality, methodology choice, or process execution. Regular analysis helps you fine-tune your approach for better accuracy over time.
Ready to take your sales forecasting to the next level? Openlead.ai provides tools to improve lead identification and outreach strategies, helping you gain a competitive edge. Get started today!